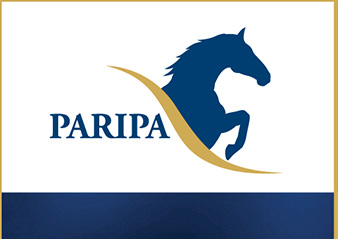
Security Operation Centers (SOCs) are one of the first lines of defenses for the computer system of an organization. Analysts are working round the clock to deal with alerts in time and filter out the real threats from the enormous amounts of data received. The pressure is increasing, they not only have to handle the already existing cases but sometimes need to predict the ones that have not yet occurred. During the years, SOCs have gained even more attention. Their role in organizations have become more important, due to the many attacks they must face day to day. However, responsibility doesn’t come without further obstacles. SOCs need to deal with even more challenges now, than ever before. Nowadays, it has become a huge problem, how to handle this enlarged number of alerts that have grown rapidly in total over the years. What is more, they have not only advanced in numbers but also in complexity and in sophistication, so they would be harder to detect by the traditional security systems. If this wouldn’t be enough of a challenge, there is the problem that there isn’t an adequate number of qualified personnel to handle these attacks. Human analysts are irreplaceable by machines, but because of the level of expertise demanded in the field, there simply just aren’t enough of them, compared to the number in which they are needed. For these many challenges it is complicated to come up with a solution that fixes everything completely. The trend that was seen in the recent years is that researchers are trying to improve the traditional systems by combining them with Machine Learning methods and thus speeding up the process of handling alerts. Machine Learning is one of the biggest hot topics in today’s world. Nowadays, everyone is using ML for various types of tasks. The base idea was that computers are able to learn to detect patterns and make decisions based on them, without being exactly programmed to do so. It is done by training algorithms on massive datasets so that they can make predictions and decisions on new data. The more the data the more precise the algorithm will be. The performance is measured by how accurate the made predictions and decisions are. With all these considered comes the question that how can Machine Learning help in SOCs? First of all, it solves the problem of manpower deficit. These algorithms can process enormous amounts of data in a much shorter time, therefore helping analyst so they only have to deal with a smaller number of cases. Secondly, because more data can be processed, it’s easier to handle the large number of data received. Analysts can only pay attention to the most important cases, that need human intervention. However, Machine Learning doesn’t solve every problem completely, therefore it shouldn’t be thought of as a magic cure for every challenge in SOCs. People need to understand what it does and what tasks can it be used for and how. Moreover, ML algorithms also need to be maintained and improved after a while to overcome further challenges that can occur in the cybersecurity field. In conclusion, Machine Learning is a great way to help out SOCs with the problems they have to face, but it must be used with insight and not as a set-and-forget method. Its many advantages can be harvested when used properly and with careful consideration. In this semester, we proposed a model that helps group nonexistent user cases to malicious or benign categories. During our work we have encountered many challenges we needed to overcome which will be mentioned and detailed in this paper.
Kapui Nikolett
2021-07-11
Támogató: T-Systems Magyarország Zrt.