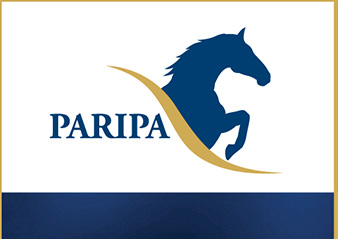
A lot of customers contact customer service by phone to solve their problems every year. Despite the high demand, call centers are frustrating and provide an unpleasant customer experience. Call centers and agents are extremely overloaded, the waiting times are high, and customers face endless loops, complex decision trees, and unexpected disconnects in today’s popular IVR systems. It comes as no surprise that businesses are gravitating towards digital assistants to improve customer experience, increase customer engagement, and decrease call center operations costs. These assistants provide conversational user interfaces, which are the most natural way to communicate with a computer program. These systems aim to solve tasks and provide information using natural language. In order to build such solutions, we have to understand natural language inputs. This has shown as a difficult task for which various solutions have been proposed using shallow machine learning and later deep neural networks. Using the machine teaching paradigm, language understanding models can be created with no expertise in statistics, probability, or deep learning. The present work can be regarded as a guideline for designing, implementing, and monitoring a conversational AI solution, using the machine teaching paradigm and various cloud-based services.
Pekár Adrián
2022-01-15
Támogató: DXC Technology