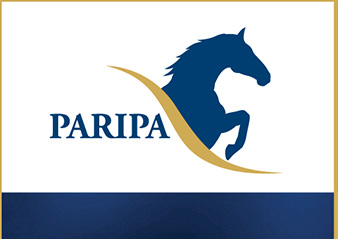
Embedded devices are increasingly connected to the Internet to provide new and innovative applications in many domains. However, these IoT devices can also contain security vulnerabilities, which allow attackers to compromise them using malware. In prior work, I proposed various binary similarity-based methods for efficient and effective malware detection on resource constrained IoT devices. In this work, I developed extensions to those that increase their robustness against adversarial samples. In particular, I studied the robustness of SIMBIoTA-ML, a recently proposed machine learning-based IoT malware detection solution against adversarial samples, proposed two adversarial sample creation strategies, and showed that SIMBIoTA-ML is robust against the first strategy, but it can be misled by the second one. To overcome this problem, I proposed to use adversarial training, and showed that it makes it possible to detect both the original malware samples and the adversarial samples with high accuracy. I also proposed a new similarity-based IoT malware detection method inspired by SIMBIoTA, but being significantly more robust than SIMBIoTA is, and showed that this method is robust against adversarial sample creating strategies that add extra bytes to an existing malware binary.
Dr. Buttyán Levente
2023-06-08
Támogató: PARIPA